Podcast Write-Up #8: Flirting with Models w/ Hari Krishnan
A word about weather futures, Mean Field Theory and whales in markets
Welcome to issue #8 of my mid-week podcast write-up series and thanks to everybody who took part in the reader survey last week, I appreciate the feedback! I'll publish the results in the coming weeks. In case you haven't participated yet and have five minutes to spare, you can do so by clicking on the link below. Thanks a lot!
Over the last two weeks, a few of my little side projects have exploded in workload and the markets have gone completely crazy with a lot more opportunities but also a higher demand for “maintenance", i.e. time to keep up. I realize I won't be able to deliver the podcast write-ups at the level of quality they deserve, so I have to suspend them for the time being. The weekend issue of fx:macro will of course be published as usual.
Here’s the latest issue of my regular weekend FX and Macro deep-dive in case you’ve missed it:
One more thing. You seem to like newsletters, so here's a great way to discover new stuff to read for free: The Sample. They will regularly send you an issue of a different semi-random newsletter you might be interested in. If you sign up using my referral link, I get bonus points and my newsletter will be forwarded to others to check out.
Release date: 08.08.2022, recorded 16.06.2022
Host(s): Corey Hoffstein @choffstein
Guest(s): Hari Krishnan @HariPKrishnan2
There were no charts in the podcast video, so every chart and link was added by me.
Notes
Hari explains his background a bit: he was brought up to be a quant without realizing it. He was fascinated by James Gleick's book Chaos (link) and its ideas on chaos theory. In finance, no one was interested in that, because no one could make money from it.
Hari's first job was helping an options market maker build floor hedging models. They were getting out of their positions or delta hedging by the end of the day on a position-by-position basis. There was the idea that you could aggregate some of the volatility risk of the books of various market makers. Hari then joined Morgan Stanley where he built asset allocation models before running a CTA himself. He also was involved in a fund-of-fund business for a while. At one point he wanted to trade for himself and not outsource things he didn't understand. Since then he's been trading macro and tail risk, long vol and currencies. He's also run an FX fund and a volatility fund for a while.
Corey asks about Hari working in weather derivatives for a while and what the similarities are between weather derivatives and more traditional instruments.
Hari explains that it's impossible to hedge in weather. You're making directional trades. They're a bit like the VIX or fixed income. There's a bit of mean reversion priced in. Weather is a bit more well-behaved than rates, but it's not tradable the same way: credit can be decomposed into forward rates, spread across countries or credit etc., while weather has a lot less of those ways to hedge or spread out.
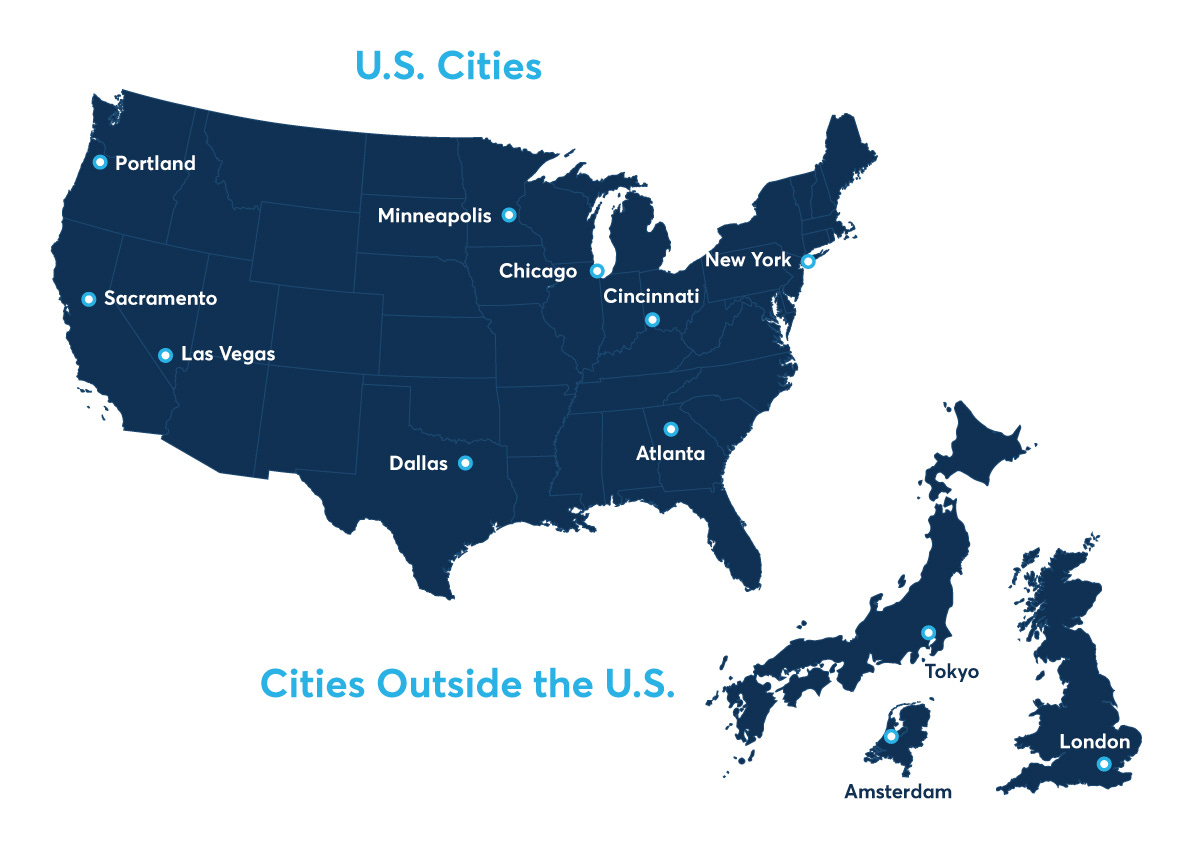
Corey asks about Hari's second book Market Tremors (link) which came out in September 2021.
Hari says he was happy with his first book The Second Leg Down (link), but the final chapter was a bit sketchy because he didn't really understand central banks and the various crisis prediction models. He mentions Richard Bookstaber's The End of Theory (link) and that he couldn't really take anything solid from it, so he wanted to write a book that looked a bit like the standard risk model with adjustments for credit and positioning risk.
Corey asks Hari to explain Mean Field Theory, the main theoretical idea from his book.
Hari explains that there's a branch in physics called Statistical Physics that deals with averages or large numbers because it's easier to look at averages than to do everything on a molecular level. An example would be temperature, and it's even in the Black-Scholes model in a sense. Mean Field Theory also applies to networks and as long as agents behave approximately the same way the average configuration is good enough.
What that means for markets is that when you look at the distribution of returns of an asset and things haven't changed then this is probably a good way to think about risk. It doesn't cover the tails or accounts for changing dynamics, but it's okay overall. But when there are major players (“mega agents”, “whales”) distorting the network, you have to modify the network in some way. So, risk can emerge when players get too large, too levered or too active. In that case, you have to model the interaction between the historical distribution and the mega agents.
If you believe a whale has to sell if the market goes down by 5% and if you can estimate the impact of that selling you can adjust your risk estimate accordingly.
Corey thinks it's like there's a return distribution that becomes conditional on the actions of market participants that have an outsized influence, and when they are forced to act it can lead to cascading effects on the potential return distribution. He wants to know how to implement that in practice.
Hari explains that you need to know who the players are. Dealers and market makers are whales. They take the other side of institutionals that like to buy puts and sell calls on equities. Dealers have to hedge actively, and if they are short options their hedging exaggerates moves, see Squeezemetrics (link) or Cem Karsan (link) for examples: if you know where dealers are short you can make a guess about how much movement to expect in those regions.
In his book, he used various statistical techniques to explain why the market was that volatile in 2020 besides the newsflow. Another topic is the ETF and ETN providers, especially around the Volmageddon event of 2018.
Central banks are also whales given the size of their balance sheet sizes and the impact of market expectations.
Corey asks: when you have a dominant agent present in markets there will always be a latent risk that isn't captured in the historical risk measurements. How do you identify those dominant players on a go-forward basis? Hari says he just asks around at banks who's buying and what big flows are going through. He's also looking at open interest and distortions of relative options prices. If put skew in the S&P 500 was too steep either the return distribution is different from his estimates or something is bidding it up structurally. An example: the IV dip in December isn't caused by structural distortions like flows as much as it is by how people think about markets.
Corey wants to know how to play defence in the presence of dominant agents. Hari's main points are
position sizing, and
avoiding overcrowded strategies like “value” or short-vol trades, especially if they have limited upside and unlimited downside.
He warns about ultra-high sharp ratios or guaranteed monthly returns. Just because something doesn't move doesn't mean it's safe.
Corey thinks the low-volatility environment in 2017 was due to a lot of dominant players were shorting volatility.
He asks Hari what he thinks about structuring tail hedges in this environment vs. 2022. Hari believes in 2006 banks were the mega players and they were suppressing volatility because it was making them money until things broke. 2017 was similar but less driven by banks. People wanted to substitute carry for alternative forms of return because interest rates weren't going to go up. In December 2021 VIX was pretty high and S&P options were quite expensive, and that was a problem for tail hedging. In the S&P hedging for him is three parts:
Gamma hedging in the short end
Spread trading for middle maturities (3-6 months)
Interest rate/Vega plays at 12+ months
The standard tail hedging idea of buying puts 20 or 25% OTM hasn't worked in 2022, because volatility hasn't gone up enough. If vol goes from 10 to 20 you can make a lot more money than when it goes from 30 to 45, because you can just buy more units of protection. Also, the market has gone down in a trend-like semi-slow way. That's why trend-following and long volatility are working well together.
Corey points out that higher levels of vol of vol have increased the sequence risk of instruments with convex payoffs, i.e. choosing when to roll precisely or when to monetize. He wants to know how to manage during periods of heightened path-dependence.
Hari says that it's very hard to use stops in short-dated options because profit is so volatile. He thinks mixing up different strikes and maturities makes sense, taking some profits along the way. Longer-dated options tend to work better with stops.
Corey asks about constructing tail hedges in rates markets. Hari says that skew in the bond market was a bit of a chameleon: when bonds were crashing higher, a call skew would form and if they were crashing lower, there would be a put skew. From 2010-19 skew was fairly priced and volatility was quite suppressed. That has changed: rates are now seen as a source of real vol.
At the beginning of the year rate hikes were beginning to be priced in in the US while in Europe no rates were being priced in. Historically, the ECB has lagged the Fed, so there was opportunity there.
Hari takes a bit of a detour and talks about Lucrezia Reichlin (link), who he's friends with, and Domenico Giannone (link… not sure if that's him, though). They developed a statistical model for estimating a GDP nowcast from economic data releases. Over time, people wanted to know why they don't have an inflation model. The model they developed doesn't predict inflation but rather characterizes the Fed's view on inflation (link). It's based on three factors:
Inflation trend anchored by inflation expectations.
Kind of a Phillips Curve factor: the relationship between the output gap and inflation
Oil prices
If the Fed raises rates there's a delay until it affects growth, and there's a longer delay before it affects inflation, especially sticky inflation. That means that the dampening in inflation may never occur. So, now there's a huge amount of inflation being priced in and then sudden and sharp episodes of deflation and vice versa. He thinks that this can be exploited in a hedging program.
Hari believes that the Fed cannot control inflation without creating a severe, unpalatable recession. It's not that the Fed is messing it up or that it's doing a great job. Rather, controlling inflation is a bit out of their control.
When inflation was low it was much easier to kick-start the economy. If the delays are as he believes then it is much easier to stimulate than to withdraw stimulation. They are a source of instability that ultimately affects how impactful policy can be.
Delays in dynamic systems aren't stable and if they change even a little they affect the outcomes in a highly unstable way. Corey asks if Hari sees delays as a source of instability not just at the macroeconomic level but also in financial markets. Hari believes there's a behavioural aspect because it takes a while for people to realize that the regime has changed. He mentions crypto where it took a long while for the belief system to develop and arguments to be formed, and then it took time for people to change their views at least temporarily and question the whole thing.
Corey mentions institutional mandates and that they have long lags. Hari says he believes that too much rigidity in hedging is not ideal.
Corey asks Hari how he thinks about monitoring the emergence or disappearance of dominant players within the options markets. He mentions the vast number of retail traders on the one side and large institutions on the other side.
Hari says that implied leverage, i.e. options, and the social network phenomenon as reasons why retail became important. Retail is important in individual names. He has a lot of bank contacts to get information about what they are doing. He thinks looking at broker chats and flows is one of the most important sources of ideas for concentration risk.
Corey quotes Hari saying “tail hedging is just a bag of magic tricks”. Hari confirms that and adds: if you have a strong directional view on the market then you don't need to tail hedge but take a directional position instead. The bag of tricks is just: what's the best hedge given today's environment. Every dog has its day: there's some scenario for any options strategy when even the silliest one pays out. Option strategies have to be regime-dependent.
He's asking: given three simple options positions (a put, a put spread, a put butterfly), what are the conditions in which each of those performs best? Asking that question is the bag-of-tricks approach.
Corey asks about machine learning. Hari answers that machine learning gets rid of the tails. There's a tradeoff you have to make: minimize bias and minimize variance, so in the end, the models always end up being long stocks and long bonds.
Great take, very interesting, thank you! ... keep it rocking! Cheers!